-
Epidemic Spreading and Peer Behavior
Conventional wisdom dictates that the most connected nodes are the best epidemic spreaders: a larger number of connections gives a node more opportunities to infect other nodes during an epidemic outbreak. Yet, contrary to conventional wisdom, I found that due to heterogeneous topology of social networks the most connected nodes are often located at the network’s periphery and fail to propagate infections efficiently. I have shown that the nodes located at the innermost network cores as identified by the k-core percolation are the most effective spreaders. My findings, published in Nature Physics brought new insights for the development of efficient immunization strategies and fueled a growing body of research on influence maximization in social networks.
Disentangling Homophily from Peer Influence. Similar to epidemics, the behavior of an individual is affected by her or his social interactions with other individuals. Unlike epidemics, however, there are multiple factors shaping one’s behavior, including peer influence, homophily, and various external factors. All these factors are strongly confounded in observational data and disentangling them requires strong assumptions about the specifics of the social process. At the core of my approach to peer behavior lies the idea that factors contributing to peer behavior can be disentangled using latent geometry of social networks: different factors corresponding to different node coordinates in the latent space. In other words, latent geometric spaces underlying social networks can be used as reference maps to track spreading processes. Tracing peer behavior using latent geometry can help one to identify geometric signatures of spreading processes, which can subsequently be used to forecast and control peer behavior dynamics. This project is funded by the NSF BIGDATA program.
|
-
Link Prediction, Soft community Inference and Network Embedding
Latent geometry offers a unique approach to the structural analysis of networks. Two nodes that are close in the latent space are similar and are likely to be connected. That is, latent proximity determines linking. If two unlinked nodes are close, then their link is either missing in the data, or they will get linked in the near future. My preliminary experiments indicate that using this general philosophy, the latent-geometric approach predicts missing and future links in the Internet and protein interaction networks, outperforming the best existing link-prediction methods. The latent geometry theory also provides a conceptually different approach to network communities: communities are viewed as groups of nodes located close in the latent space. This approach does not suffer from many difficulties plaguing traditional methods (e.g., uneven community sizes, overlapping communities, unknown numbers of communities, and resolution limits) and explains remarkably well a series of peculiar properties of the community structure in the Internet.
From the practical perspective, in order to predict missing links or to identify network communities, one first needs to map (embed) the real network of interest to its underlying space. Together with my colleagues, I developed a series of network embedding methods based on the maximum likelihood estimation (MLE) and showed that they accurately infer the node coordinates in hyperbolic spaces. It has recently been proven that MLE in latent-space network models is guaranteed to infer correctly the latent-geometric coordinates of nodes in large networks. However, running times of algorithms that implement MLE exactly, such as Markov Chain Monte Carlo methods, tend to be unbounded. In an ongoing project funded by the ARO I am developing scalable approximation algorithms for MLE-based embedding methods in hyperbolic spaces and generalizing them to weighted and multipartite networks.
|
-
Network Resilience
Our society’s critical infrastructure lacks an ability to sustain its functionality following adverse events, such as epidemics, natural disasters, and cyber threats. Growing complexity and uncertainty associated with upcoming threats make the application of traditional risk-based analysis methods prob- lematic. In the light of the above challenges, the optimal course of action is to build resilience into complex interconnected systems. To this end, I am working on defining and testing resilience metrics on different network architectures (joint work with US Army Corps of Engineers) and designing resilient communication protocols in technological systems, such as greedy geometric interdomain Internet routing (joint work with CAIDA and Simula Research groups).
|
-
Signaling Patterns in the Brain
The brain is the center of the nervous system that exerts control over all bodily organs: it accepts input signaling from other organs, processes it, and routes the processed information to the appropriate destinations. To this end, the brain can be regarded as an information processor. Yet, information routing in the brain occurs without any global intelligence. There is no centralized authority within the brain dictating what information is sent where.
How can the brain execute such routing then? How efficient and robust is it performing this routing task?
A plausible explanation is that signaling in the brain is driven by the geometry of its latent space. Even though anatomic regions of the brain may not know the entire connectivity state of the brain, signaling in brain is akin to greedy geometric routing: in order to execute certain functions, neural signals are preferentially propagated towards the regions responsible for this function using the latent geometry of the brain. While the terminal goal of this project — understanding how information is processed by the brain — is unattainable in the near future due to the lack of small scale connectivity and signaling data, in the initial phase of the project I aim to (i) uncover the latent geometric space underlying the brain and (ii) use resulting latent geometry of the brain along with available functional data to propose and validate candidate geometric mechanisms for signaling in the brain.
|
-
Molecular Mechanisms of Human Diseases
As a postdoctoral scientist at the Center for Complex Network Research (funded through the NIH CEGS program) I studied molecular mechanisms of human diseases. Diseases often result from the abnormality of multiple molecular processes taking place in human cells. Relationships among these processes are encoded in the interactome, a network integrating all physical interactions within a cell, from protein-protein to regulatory protein - DNA and metabolic interactions. The molecular components associated with a particular disease are believed to interact preferentially among themselves, forming an interconnected subgraph or a disease module within the interactome. In a series of works I demonstrated that disease genes display a statistically significant tendency to agglomerate in the same neighborhood of the interactome and developed rigorous techniques to evaluate network-based separations between disease modules.
Deciphering Molecular Mechanisms of Human Diseases. The identification of disease modules is only the first step toward a systematic understanding of the molecular mech- anisms underlying a complex disease. Subsequent steps include deciphering unknown components of a disease and understanding disease-disease and disease-drug relation- ships, with the ultimate goal of developing novel drugs and/or repurposing existing ones. Despite their direct nature, traditional graph theoretic methods used in systems biology are rather limited. These methods rely on the network-based distances between nodes. Most biological networks have a very small topological size and can be traversed in a few steps. Thus, the range of possible distances to work with is limited to a small number of integer values. More sophisticated network-based separation measures, on the other hand, are often plagued by a loss of triangle inequality and are biased in the case of incomplete data. At the heart of my approach lies the latent geometry theory. Within the approach proteins correspond to points in the latent space, chosen to minimize distances between interacting proteins and at the same time to maximize distances between proteins that do not interact. Resulting coordinates can be used to identify missing links, detect disease modules and quantify their effect on biological processes. While the latent- geometric mapping of the human interactome is currently underway, my preliminary results obtained for the interactome of S. cerevisiae allow for detection of functional protein modules and prediction of missing interactions. This project is partially funded by the Army Research Office.
|
|
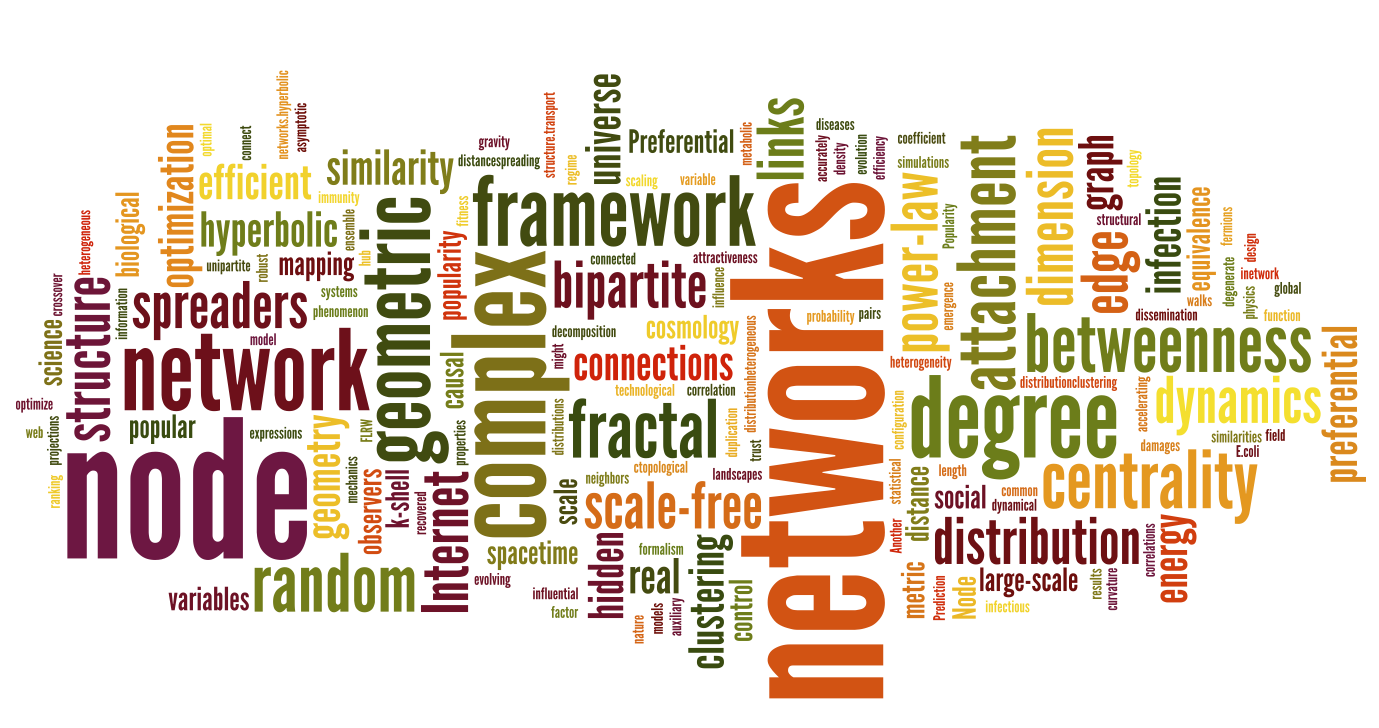
A word-cloud
of key-words related to my research, via Wordle
|